Every day, NIQ empowers retail and manufacturing clients across 90+ countries, 1.4M+ stores, and trillions of records with artificial intelligence (AI) and machine learning (ML) to drive actionable insights, beginning with product innovation.
With the highest quality raw data, common key data sets, trustworthy analysis and modeling, and quality control supported by human intelligence, NIQ is the premier partner to today’s top manufacturers and retailers.
In this report, our AI experts at NIQ BASES discuss the democratization of Generative AI and its implications for CPG product innovation.
Drawing on their decades of experience building patented AI solutions, they outline how manufacturers can take a positive, practical, and purposeful approach to their own AI exploration.
The democratization of artificial intelligence
“If you’re an AI novice, worried you’ll never catch up with those who got a head start, there’s good news: the rapid evolution of this technology is continually leveling the playing field. If you’re already an AI pro, don’t get too comfortable — because the rapid evolution of this technology is continually leveling the playing field.“
Ramon Melgarejo, Managing Director, NIQ BASES and Global Analytics
The fast-paced evolution and widespread adoption of generative AI (GenAI) is paving a path of disruption through a number of industries. The latest models have become more advanced and easier to operate — and you don’t need a PhD in computer science to use them. Democratization of access along with rapid improvements in scalability, reasoning, and quality of output have made the latest AI models more effective and pervasive than ever before, with far-reaching implications across many industries and business practices — including how we innovate.
In this report, we examine how GenAI can revolutionize your product innovation processes, discussing how it works and its potential to enhance creative and strategic processes. We’ll also tackle the associated challenges and opportunities, equipping your organization to successfully harness this emerging technology.
To understand how GenAI will change CPG innovation, it’s first helpful to understand how it works. Large Language Models (LLMs) like ChatGPT are trained on huge volumes of text and, when asked a question (the prompt), generate the answer one word at a time.
These sequence-to-sequence models make logical predictions, choosing the word that’s most likely to follow based on learned patterns from the training data as well as the prompt that the user has input into the system. This makes the output contingent on the quality of the training data and the prompt.
The ability of generative LLMs to understand and interpret natural language, synthesize huge amounts of input, and quickly generate text and content creates an opportunity for innovators to do their job better and faster.
GenAI applications span several areas, each requiring different levels of rigor and investment.
Some initial applications to consider for your product innovation process:
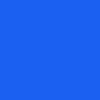
Data analytics & interpretation:
- Summarize qualitative research
- Conduct sentiment analysis on research and reviews
- Simplify the interpretation of both qualitative and quantitative data
Idea & concept generation:
- Generate innovative ideas and brainstorm with GenAI chatbots
- Develop product ideas into comprehensive concepts
- Explore various combinations of benefit claims and formats
Other advanced applications:
- Extract new insights by mining multiple past research
- Utilize advanced GenAI and rich prompting for efficient surveying with virtual, synthetic respondents
GenAI’s ability to canvas and synthesize information is already helping manufacturers jumpstart the creative process, whether it’s brainstorming new concepts, expanding on ideas, or fine-tuning product details and messaging. When used effectively, GenAI has the potential to accelerate product innovation cycles, pulling from a vast repository of cross-category insights that help manufacturers come up with their next innovation even faster.
“We’ve seen remarkable progress with the capabilities of these models, even from only five years ago. Yesterday’s models were difficult to customize with our own research and expertise and generated less than stellar output. Today, we’re conducting our own in-house experimentation to develop solutions that will help accelerate the innovation process — with promising results.”
Mark Flynn, Head of Product, NIQ BASES
Which means it’s unlikely that AI will replace innovators’ jobs — instead, innovators who embrace AI as an enabler to do their job more efficiently and effectively will be the ones who flourish.
But as with any new business initiative, before diving headfirst into the GenAI landscape to develop your next great innovation, you must first lay a solid foundation to ensure your organization is set up for success.
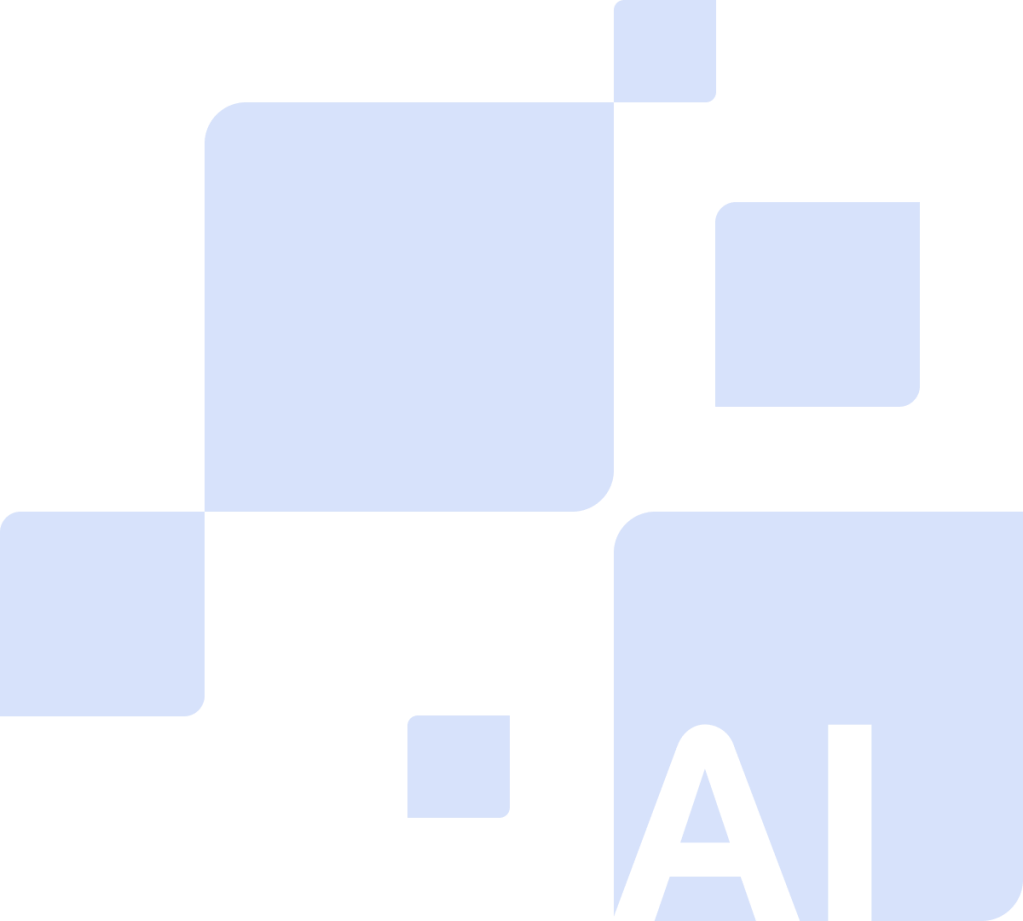
Recipe for success:
How to make AI work for your organization
GenAI holds promise for the CPG product innovation industry, and businesses who take steps to implement proper guardrails should not be afraid to embrace it as part of their toolkit — even with its often-publicized challenges and pitfalls. Every emerging technology comes with rules for the road: For example, the microwave oven was a revolutionary innovation that helped people cook or heat their food in record time. But it came with a learning curve (as anyone who ever tried to reheat their coffee with a metal spoon in the cup realized the hard way). No one abandoned their microwaves — they simply learned when and how to use them most safely and effectively.
Let’s explore how to leverage the benefits of GenAI while mitigating the risks so you can get the most of your technology investment.
Getting started with GenAI:
Lay the organizational foundation
Before exploring AI opportunities and applications, get your leaders on the same page by establishing a dedicated task force that aligns teams across your business. This should be a multi-disciplinary group with subject matter experts who go beyond tech and data science. For instance, your legal team can help you assess intellectual property (IP) and other legal risks, your data security team can ensure the correct steps are taken to protect company data and IP, and product teams closer to your end users can provide a customer-centric perspective.
Everyone has a role in GenAI
AI task force responsibilities can include, but are not limited to:
1
Establishing guidelines and protocol for AI ethics and data security
2
Determining AI use cases and applications across the business aligned with your company’s overall strategy, goals, and objectives
3
Determining when solutions should be built in-house or by third-party suppliers
4
Determining how suppliers (current and new) are allowed to use your data in AI models
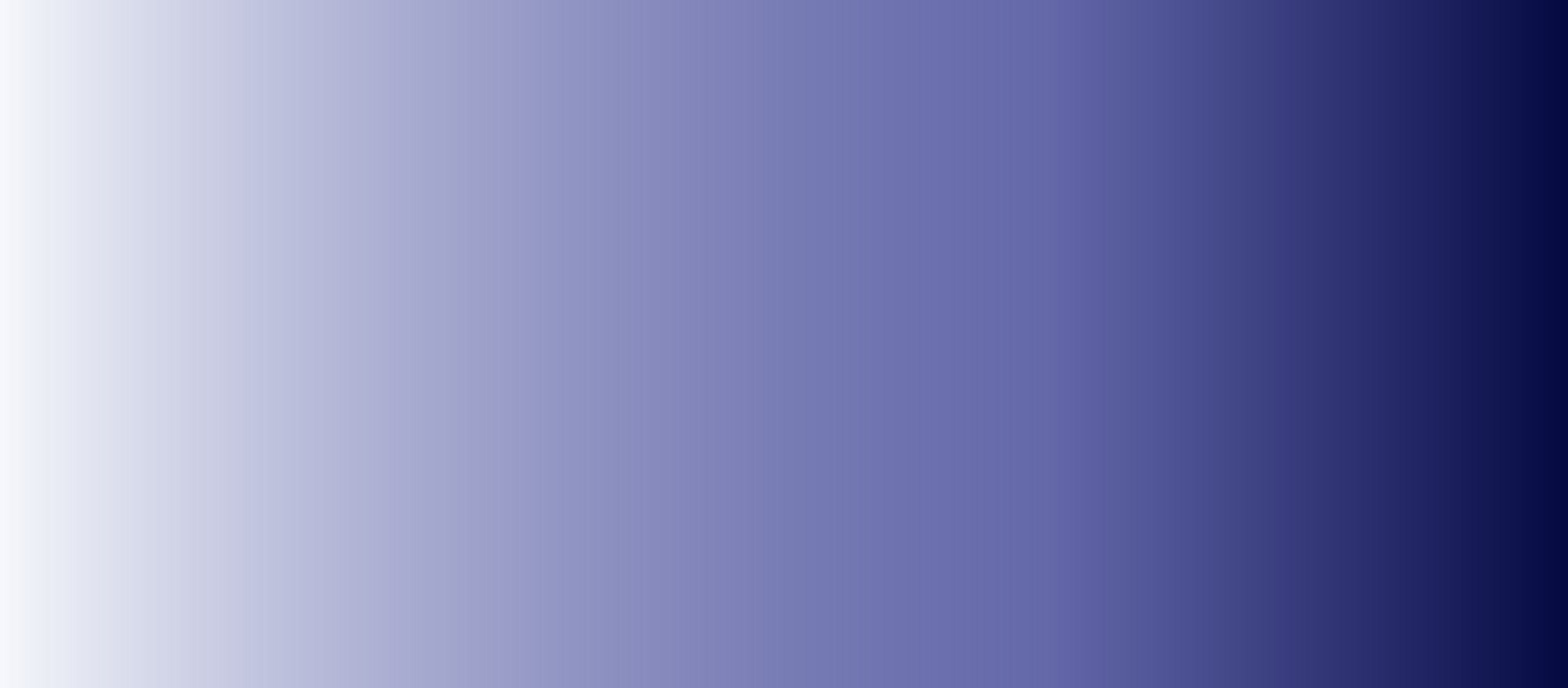
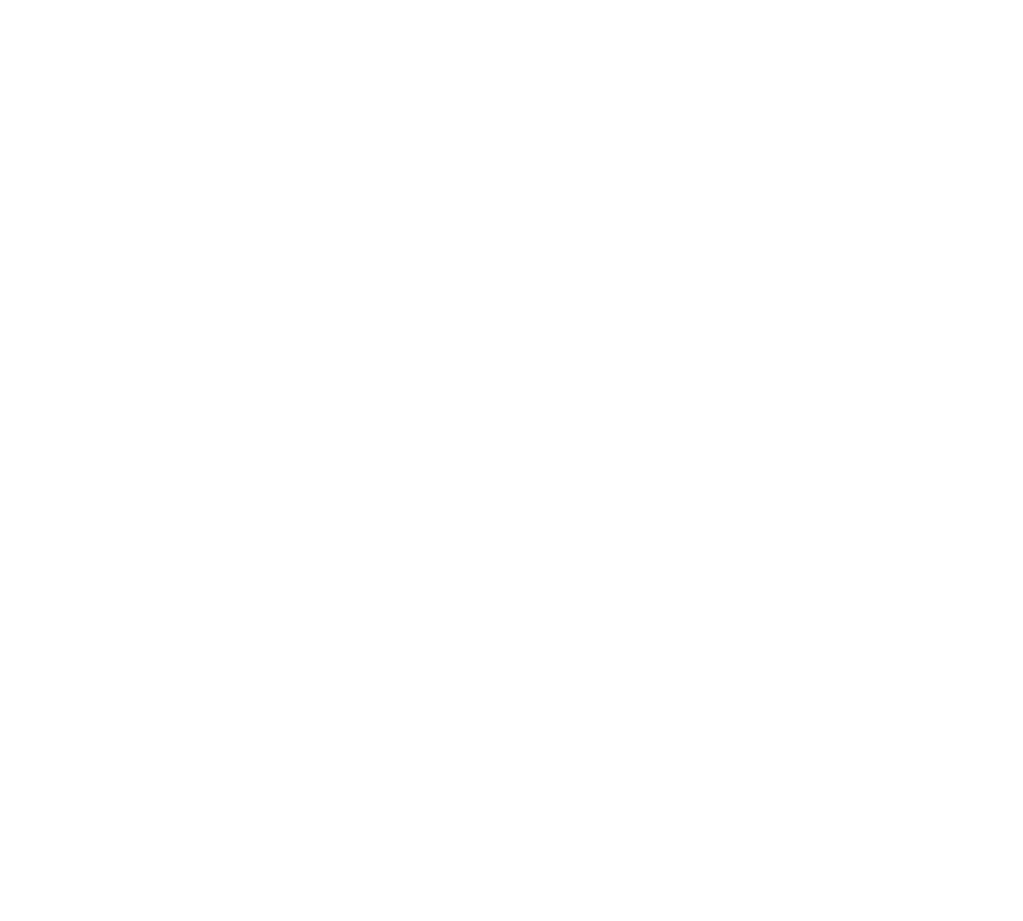
Make the most of your AI task force
The function of a core, multi-disciplinary AI team may change over time. Once best practices are rolled out across the organization, they should become business as usual. Your task force, however, should remain in place to stay ahead of emerging technology, discover new use cases, and prepare for the opportunities and challenges it brings.
At NIQ, one of our own evolutions was integrating key leaders on our AI task force into NIQ Labs — our innovation center dedicated to tackling our industry’s greatest challenges.
Want to learn about our AI exploration in the product innovation space?
The following questions can help guide your AI task force toward solutions that are safe, effective, and a good fit for your business needs.
Establishing the use case:
Do I even need GenAI to solve this problem?
Many companies are jumping onto the GenAI bandwagon simply because the technology exists and everyone seems to be using it. This phenomenon is not a new one: A 2019 survey by venture capitalist firm MMC found that 40 percent of European startups that are classified as AI companies don’t actually use artificial intelligence in a way that is ‘material’ to their businesses.
With so many businesses rushing to capitalize on GenAI’s popularity, organizations must perform their due diligence in vetting potential vendors, not only on their application of AI, but whether they’re truly using AI at all.
Don’t let hype drive your decision making. Instead, ask yourself: What problem am I trying to solve? And what’s the most direct, simple, and straightforward answer to this problem?
In many cases, a statistical or other, non-generative machine-learning model may be more appropriate. If you’re summarizing comments from your customers, GenAI is a great solution. But if you’re trying to forecast sales or use it for data extrapolation, machine learning and regression toolkits might be a better fit.
And even certain use cases, like text creation, must be followed up with validation. GenAI is very good at summarizing information you provide because you can control the input and how you want it summarized. But if you want GenAI to create new information for you, your AI team must have the data to verify whether the information the model provides is accurate.
So don’t forsake your current analytic tools that are already working well. Instead, explore what new use cases GenAI opens for you to innovate better and faster.
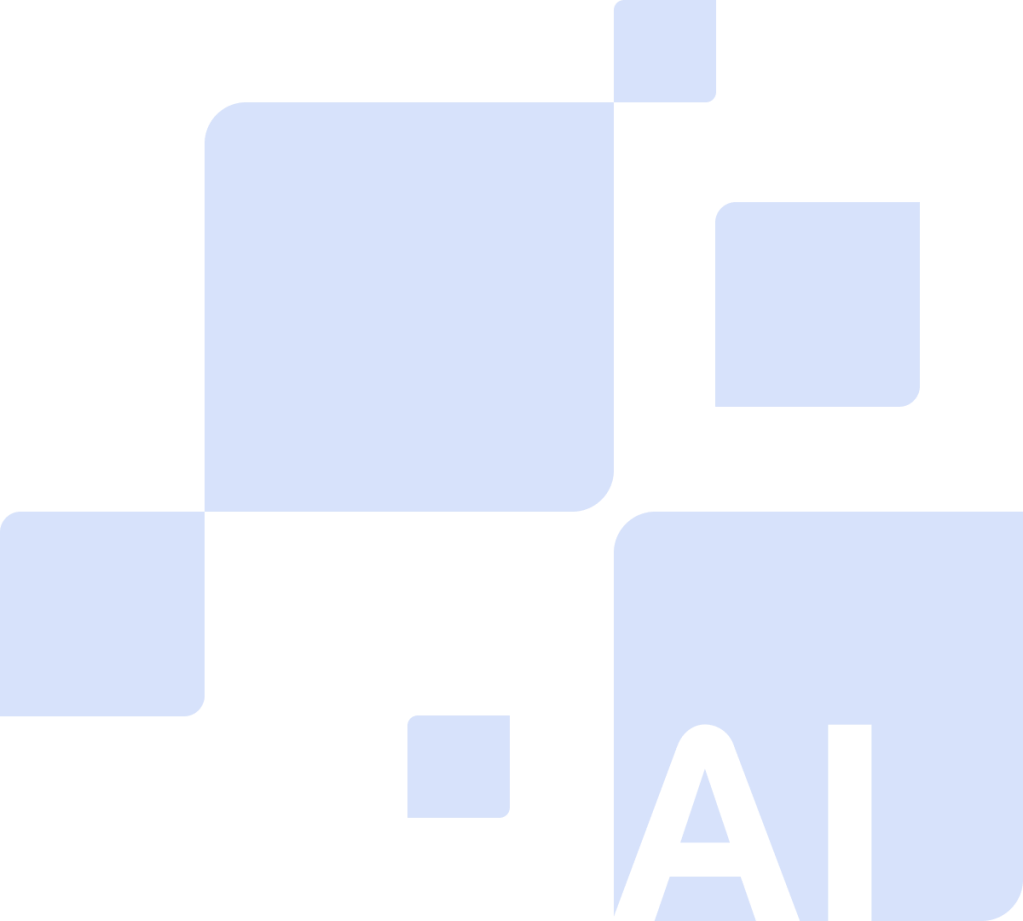
Partnering with trusted experts:
What should I ask before investing in new applications for GenAI product innovation?
Now that you’ve established your use case for GenAI, you’re ready to explore solutions. Asking the right questions allows you to make educated decisions so you can get the most out of your technology investment and keep your data safe.
Just as you would vet other types of R&D modeling research, you’ll apply similar standards and best practices to AI investments. The following questions can help you find the right fit.
How do you balance accuracy and scalability?
Ideally you would build models that are incredibly accurate and easy to scale to new use cases, geographies, and trends. However, it’s more likely that you’ll need to make tradeoffs when picking the right GenAI investments.
Generalized models have become so advanced that you probably won’t need to build a model from scratch — your AI team can use domain-specific training to customize the model to your use case so you can apply accurate, relevant industry data to solve your problem.
Technically, you can customize a model with any type of data — but is this worth the time and cost? Ultimately, it requires a balance between accuracy and scalability.
For example, perhaps you have a certain tone that you’d like to use in your ad copy, so you’d like to use a custom-trained LLM to give you an output in that tone. But what if you work across several different brands and countries? Would the tone for ad copy promoting laundry detergent in the US be the same for promoting haircare in Japan?
While you might be tempted to ask your AI team to train separate models for each of the brands and countries that you manage, this would become time- and cost-intensive, resulting in diminishing returns. You may get a slightly more final product in doing so, but you’d likely lose the efficiency that you were hoping to gain through using an LLM in the first place.
When investing in these models, you’ll need to make conscious decisions about whether it’s worth customizing to your exact use case instead of using more generic models and then curating how you input information and utilize the outputs.
Is this model easy to update?
Can it keep up with the latest technology?
GenAI technology is evolving quickly, which means today’s model could be outdated by next year. You may also be working with data that gets updated frequently. These factors should play a role in how your AI team chooses to train your model.
One method is fine-tuning, which involves training an existing model with your unique datasets. However, this is a time- and cost-intensive process, and as technology or datasets change, you would need to rerun the training — making fine-tuning a less-scalable, less-nimble option for many use cases.
An alternative to fine-tuning is rich prompting, which provides enough information and instruction in the prompt itself (rather than in the model training). As data is updated, your AI team needs only to adjust the prompts rather than re-train the model from scratch.
How might this work in practice? Suppose you’re a baked goods company with new primary research on wine and chocolate pairing trends. You might design prompts inclusive of that research, as well as specific category knowledge, your brand strengths, and organizational goals, versus retraining the entire model for each data fluctuation. In this case, and others that work with continuously updated datasets, rich prompting is the better approach.
How do you protect against bias and hallucinations?
How do you validate output accuracy?
When experimenting with GenAI, be aware of how bias and hallucination can affect the quality of your output. In addition to developing protective guardrails against these phenomena, your AI team must also implement validation processes to ensure output accuracy.
Bias in language models refers to the tendency of a model to generate outputs that reflect the biases present in the training data it was built upon. These can manifest in the model showing preference for or against certain demographics, ideologies, or topics.
As advanced as GenAI models have become, it’s easy to forget their output is only as good as their input. No matter how human the responses seem, LLMs don’t discern information in the same way humans do. If an incorrect pattern was in the training data, it can lead to incorrect outputs. Factors like flawed data sampling, data that reflects implicit bias, and data that reflects historical inequities can lead to bias in outputs.
GenAI models can be prone to different types of bias
Implicit Bias
Unconscious associations or attitudes toward certain groups of people. Because GenAI models learn from their training data, they inherit any human, implicit biases that are present in that data — such as racial, gender, cultural, or generational biases.
Popularity Bias
Because models like ChatGPT are trained using the internet, the frequency or popularity of information can affect output — even if it’s misinformed. For example, we know from our work on innovation vitality that the 95% innovation failure rate is a myth. Yet a number of articles can be found on the internet asserting its veracity. If a model is trained on these articles, it will likely quote that high innovation failure rate when prompted, given the sheer amount of misinformation on the topic in its training data.
Recency Bias
If the content you’re asking for requires recent knowledge (such as new trends or current events), the models may not yet have the relevant information in their training set. Innovators must leverage primary research to fill the gaps in the model’s knowledge.
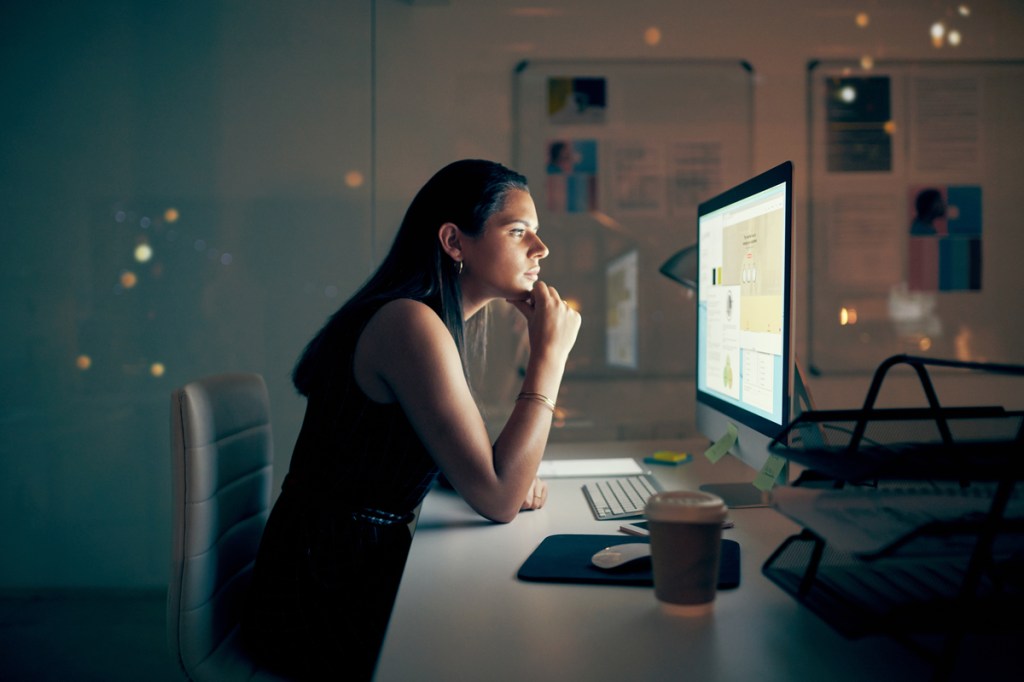
LLMs make logical predictions for each word, choosing what’s most likely to follow based on learned patterns from its training data. But they have the potential to generate inaccurate or nonsensical outputs — a phenomenon called “hallucination.” Because these responses are conveyed with confidence, they appear to be true and are therefore difficult to detect.
Hallucination is when a language model produces information or data that is not grounded in reality or factual accuracy, effectively “making up” details that may sound plausible but are not true.
The risk of hallucinations can vary according to use case. For example, GenAI used for text-based data summarization tasks is less likely to hallucinate, but if it’s generating new information for you — like new benefits or claims — you must validate the outputs to confirm accuracy. Similarly, with the latest enhancements in technology, users can call upon certain functions to execute numeric data analysis, but using LLMs directly for complex numeric data analysis is not recommended.
Indeed, the technology is rapidly advancing, as evidenced by the 40% improvement in generating factual responses between GPT-3.5 and GPT-4. However, a cautious approach is crucial. Inquire whether and how your AI team can provide data to verify the accuracy of their outputs. Reliable data sources, primary research for validating AI outputs, and the capability to constantly monitor accuracy are industry best practices. If you are unable to verify whether your model’s output is correct … should you be using it?
How will our data be stored?
Will our data be shared with a third party? How will it be used for training future models?
Protecting your data and IP is critical when using GenAI applications. You likely already understand the importance of protecting against unauthorized access to your data. However, you may not be aware that you could actually be providing access to your data when you use GenAI or work with vendors to build AI models.
There are a number of different ways to access GenAI. In fact, there is a proliferation of vendors producing plug-ins and other types of easy interfaces that allow users to simply paste in data and receive something back — but it’s not always clear what the guidelines and privacy considerations are. Using a free, open source LLM, like ChatGPT, means anything that you input as a question or prompt can potentially be used for future model training. In fact, Google DeepMind recently published a paper about its ability to extract training data from ChatGPT, raising new questions about data security.
Without due diligence, the information you share with an open source LLM — including that proprietary new concept your team has been developing — could ultimately be provided as part of an answer in a future model to anyone, including your competitors.
To protect your information, we encourage investment in safe ways to leverage GenAI — for example, through a premium membership, or a “walled garden,” which contains your information and keeps it inaccessible to the public or any other third-party. Be sure to ask potential vendors how your data and the information entered into prompts are being stored, shared, and used.
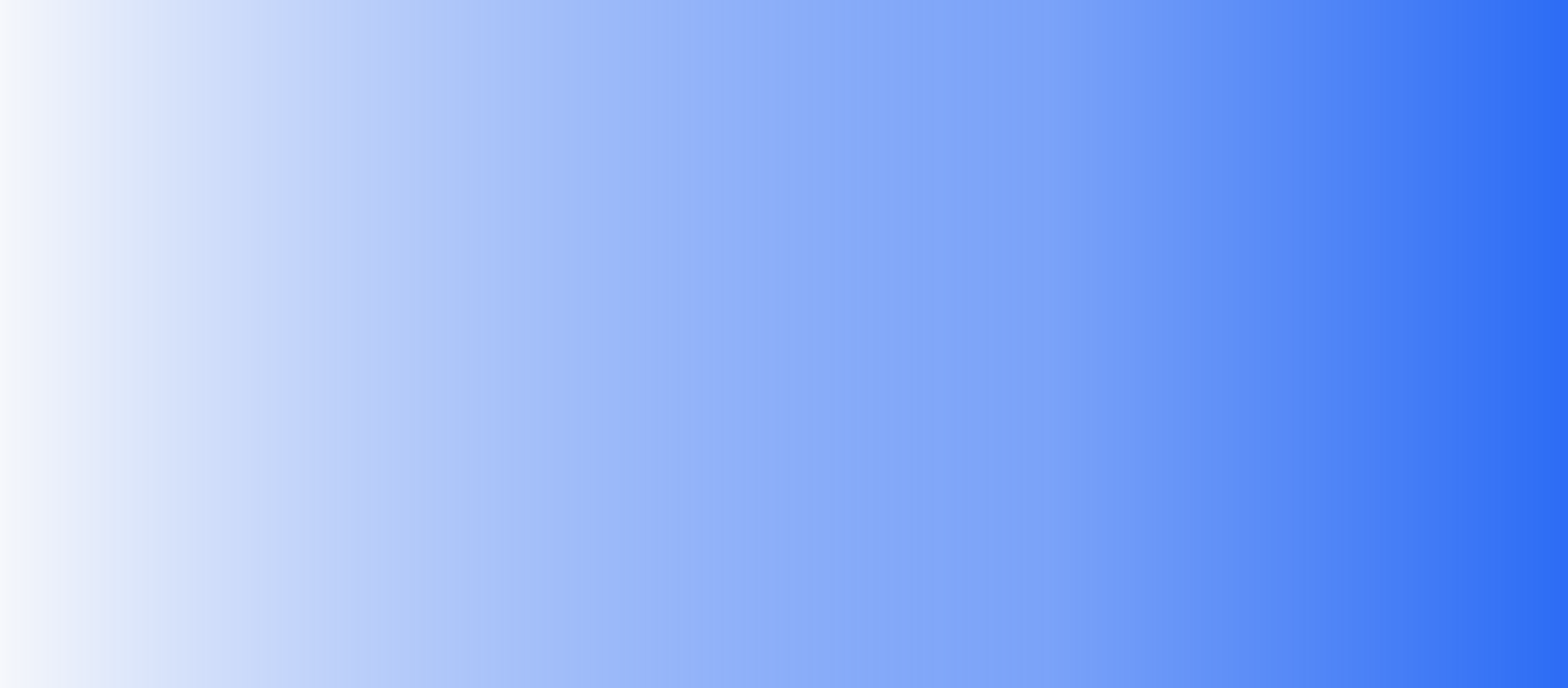
Differentiating from competitors
If everyone is using GenAI for product innovation, what’s my competitive advantage?
Now that you have the right models in place for your use case, along with protocols to validate outputs and keep data secure, how do you use these tools effectively?
While GenAI can support innovators across a variety of tasks, let’s explore how it can help you jumpstart idea generation. After all, it’s not the tool itself that will unlock true breakthrough product innovation — it’s how it’s being used.
Up your product innovation game with GenAI by leveraging:
Clear prompts
Provide specific instructions with additional context, objectives, and desired outcomes clearly stated
Foundational research
Leverage unique, trusted data sources to get the most out of your GenAI investment. Remember: Your outputs are only as good as your inputs!
Innovation expertise
Keep humans in the loop; GenAI is a tool to augment — not replace — innovators’ expertise
If you simply prompt the model to come up with a great product idea, you’ll probably come up short (mosquito-repellent roast potatoes and cookie-vegetable stir fry aren’t likely best sellers). Similarly, if you put in a generic prompt, you’ll receive an equally generic idea. However, if you craft a specific, insightful prompt leveraging foundational research, then you can enable the model to pull together a unique combination of innovative and interesting ideas. Let’s illustrate with an example.
A manufacturer wants to create new, innovative packaging for dish soap and hopes to generate ideas by comparing packs in other categories. LLMs can manage this kind of analogical thinking with the right prompts, but at a much larger scale. With a vast repository of data, it has the ability to make infinite combinations across industries and categories without getting tired. What if the manufacturer asked the LLM to generate dish soap pack ideas inspired by breakfast cereal boxes? Or ice cream cartons? By combining the right prompts using foundational research — for example, your brand strengths, personas, and category knowledge — even seemingly off-the-wall comparisons can lead to interesting insights, which you can keep running in the prompts until you’ve struck gold.
Note that this exercise is meant for using GenAI as a tool for ideation and inspiration, not for exact outputs or fully-baked ideas — especially when pulling from open data repositories like the internet. Remember that, on its own, an LLM doesn’t come up with truly novel ideas. In addition to some of the aforementioned watchouts about bias and hallucinations, using exact outputs also risks potential intellectual property conflict. An important role of your AI task force will be to monitor the latest legislation around this topic and determine best practices for effectively using GenAI
Ultimately, GenAI will change how you innovate — but it won’t do all the work for you. For now, it’s safer — and more effective — to use it as a tool for inspiration with honed prompts that play to your strengths and help differentiate you from the competition.
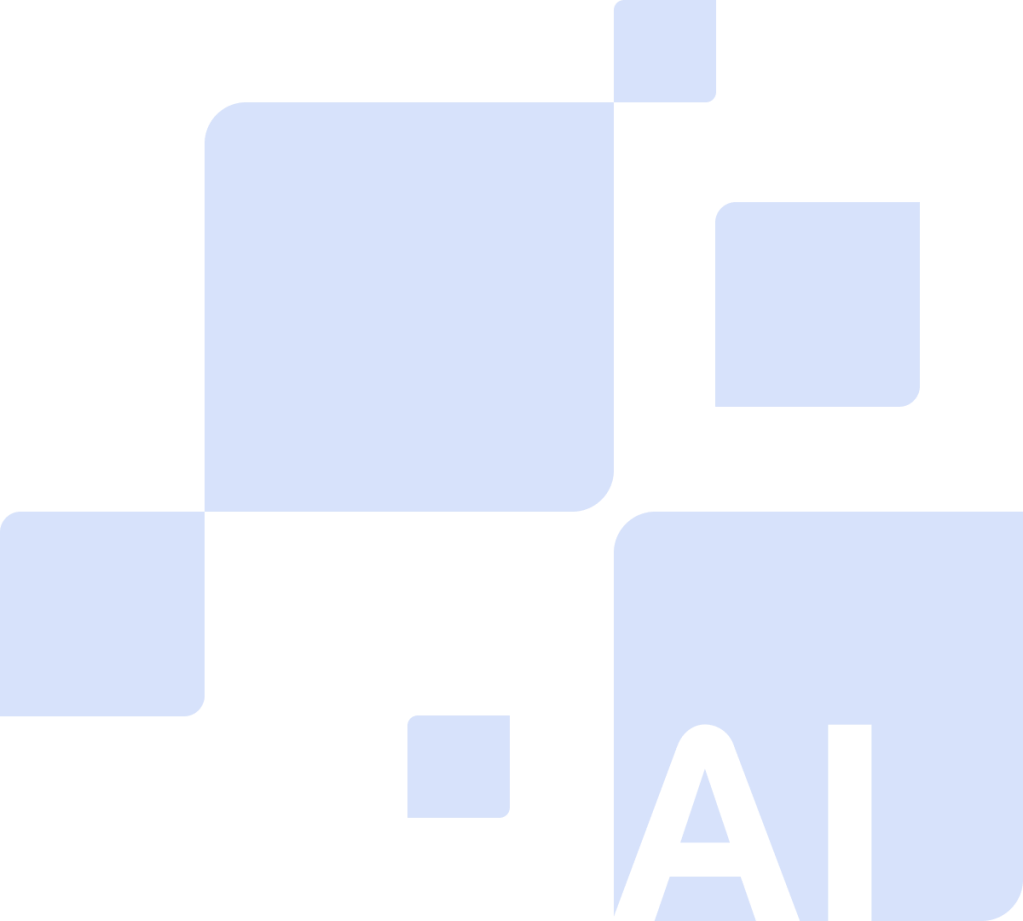
GenAI will change the game
In just this past year, new GenAI technologies have enabled capabilities that even field experts expected to be years or even decades away. The pace at which these models have evolved also makes it clear that predicting their full potential is not only challenging, but also, perhaps, a futile endeavor. However, it’s unmistakable that these technologies will significantly influence the landscape of product innovation, branding, and market research.
As we have seen, GenAI is not just an incremental advancement; it represents a paradigm shift in how we generate ideas, conduct research, and measure performance. Given the rapid evolution and increasing accessibility of these technologies, it’s imperative for companies to not just acknowledge but actively embrace AI in their product innovation strategies. Those who do will find themselves at the forefront of industry, benefiting from accelerated innovation cycles and deeper, more nuanced market insights. On the other hand, companies that hesitate or ignore these advancements risk falling behind in an increasingly competitive and AI-driven market landscape.
While the full extent of GenAI’s applications may be unknown, its critical role in shaping the future of market research and CPG product innovation is undeniable. With proper guardrails in place, companies across all sectors should proactively incorporate AI into their strategic planning, ensuring they remain agile and competitive in a rapidly evolving digital era.
NIQ’s first GenAI-based tool, “NIQ Ask Arthur,” is integrated into our Discover platform and empowers users with AI-assisted global search and personalized recommendations based on KPIs and always-on analytics. Offering AI-generated insights within reports, the tool simplifies complex data interpretation and facilitates informed decision-making.
Want to learn about our AI exploration in the product innovation space?
Many thanks to our report contributors:
Julie Dellert, Lauren Hirs, Aritra Kanjilal, Martin Levanti, Kamal Malek, Noelle Salerno, Courtenay Verret