The importance of survey research
Consider the last time you stayed in a hotel, had your car serviced or even picked up your curbside grocery order — did you receive a survey asking about your experience?
Surveys have been the underpinning of the market research industry dating back to the 1920s. In many ways, the survey industry is stronger than ever: An estimated $1.1B is spent on survey software in the US every year. Yet as the accessibility to and prevalence of surveys increase, so has the paradoxical concern about their validity—and this may especially be true for surveys designed to be used for predictive analytics. This skepticism, in combination with increasing pressure to make business decisions more quickly and economically, has companies exploring a proliferation of new—and old—alternative methodologies.
Does this mean the sun is setting on survey-based research? Not so fast. Let’s explore some of the emerging doubts about this popular methodology, industry efforts underway to address these underlying concerns and best practices for interpreting data claims and translating them to human behavior.
Is survey research falling out of favor?
Given that survey-based research is such a well-established way to collect data and inform decisions, recent negative sentiment has caused us to reflect on why these doubts are surfacing.
We have some hypotheses: First, there have been some well-publicized failures in the survey industry—election polling being one very prevalent example. It is also likely that some companies using surveys have encountered misleading insights themselves. Then, there is the bestselling book, Everybody Lies, which draws into question whether you can really trust what people tell you in survey research.
Finally, there is the unmistakable influence of the internet, where companies are exploring new ways to run online test markets and other transactional and behavioral experiments on e-commerce and social media platforms. Some argue that these behavioral experiments can be trusted more than surveys and that the digital ways to observe behavior allow them to collect data and insights with more speed and agility, just as the tech sector has successfully employed with its new product development. These are all compelling reasons to look at the efficacy of survey-based research with more scrutiny.
Do people really lie in surveys?
Let us start with the question of whether survey responses can be trusted. As the aforementioned book contends in its title, when it comes to surveys, everybody lies.
Is that true? Given that our own company largely relies on survey research, you may be surprised to hear our answer: We agree with this assertion—sort of.
At NIQ, we are in the unique position of having a wealth of survey data on consumer intent, in addition to behavioral data on what they actually purchase. From these insights, we know that one cannot take a person’s survey response at face value. However, we find that although survey respondents typically overstate what they do or will do, their claims in fact have a clear relationship with actual behavior that is informative and predictive.
Look at Figure 1 below. You can see that respondents claimed they would buy far more units of product than they actually did. Nevertheless, as the number of units people claimed they would buy went up, so did the number of units people actually bought in-market. In other words, the survey claims in this example were, in fact, very indicative of which products would generate the most units purchased per occasion.
A similar dynamic where survey claims give a clear indication of actual in-market behavior can be seen in Figure 2.
Although survey claims do not exactly match actual behavior, the brands consumers claimed to purchase most are those that consumers actually did purchase most in-market.
The key to using survey research effectively is having the knowledge to accurately interpret these claims and how they will translate to real behavior.
A simple concept, that is not so simple
The premise of survey research is simple: If you want to know what people are thinking or doing, just ask them.
But, as we just established, you must be able to account for overstatement or, in some cases, understatement in order to interpret what people truly mean. And while this concept might seem straightforward, as you can see in Figures 3 and 4, there are many factors—like demographics, cultural preferences and even geographic location—that influence how much people overstate, which makes data interpretation complex.
How survey companies and suppliers build and engage with their panelists also makes a difference. This includes how much respondents are compensated for taking the survey and the length of time they’ve been on the panel (see Figure 5). Additionally, survey sample companies employ a variety of techniques to enlist participants, and these methods also influence scores (see Figure 6).
We have observed these dynamics on the panels we own and manage, as well as on the external panels with whom we partner.
The implication is that you can get different survey scores depending on how data is collected and managed. See Figure 7 for a more compelling illustration of this dynamic. Here, we tested the same three concepts with consumers who were recruited by three different methods.
The three methods produced very consistent yet very different results, depending on the lens through which you view them.
In terms of consistency, all three methods show that the fruit snack concept generates far more interest than the toothpaste concept, whereas the cheese snack concept generates the most interest out of all the concepts. So, if the goal of this study was to determine which of the three initiatives generates the most interest, all three data collection methods would result in the same conclusion.
On the other hand, if we look at the absolute scores, you can see that they are very different across each of the data collection methods. Thus, if someone were to look at the raw scores alone to determine the absolute level of appeal for each product idea, they would likely have a very different takeaway.
For example, all three product ideas look to generate very high scores with Social Media Recruiting Method B; however, this is due to high levels of overstatement in that recruiting method. To conclude that the high scores indicate consumers truly find all the product ideas extremely appealing would be a mistake.
Another problem arises if you are testing ideas iteratively and using different collection methods from project to project—something that is not uncommon, given increasing speed and capacity requirements in data collection. Under this scenario, you may draw incorrect conclusions on whether you’ve improved the appeal of the idea in the latest iteration, as change in scores could be driven by the data collection method more than true changes in consumer appeal.
For someone to draw an accurate conclusion from this data, they would need to have the right understanding of how much overstatement levels vary across each of these data collection methods.
Figure 8 illustrates another example of what can happen if all the variables that impact overstatement levels and data stability are not being appropriately managed in research.
As a R&D exercise, we tested the same concept multiple times over a period of many weeks using three different panels: our BASES-owned panel and two external panels. As the chart illustrates, the BASES panel, for which we controlled all the important variables, produced very stable scores; as a result, one would come to the same conclusion around the potential of the initiative regardless of which of the five tests they were referencing.
In contrast, we allowed the external panels to manage the sample as they typically would, and the consumer scores showed much more variability from test to test. The implication is that one could come to a very different conclusion about the potential of the product depending on which test they are looking at. Also, as we mentioned in our Figure 7 commentary, this type of test versus retest variation creates problems for iterative testing. Those who are trying to be agile but not using the collection methods that deliver stability could be getting a false signal of improvement and inadvertently making their ideas worse. The problems outlined in this example underscore why suppliers must control the variables that influence scores.
Getting survey data right requires companies to heavily research the dynamics that influence scores and overstatement levels, model for them when constructing database norms and predictive models and control them from study to study. Yet many overlook them, ultimately delivering subpar results in the validity and predictability of their research. It is possible that these examples of shoddy research account for some of the recent pushback toward survey data.
The need for speed
Last summer, “Top Gun: Maverick” set an all-time record for box office sales on Memorial Day weekend in the US. Afficionados of the original Top Gun movie might remember one of Tom Cruise’s most famous lines: “I feel the need…the need for speed.”
This mindset isn’t just for elite fighter pilots—it sums up the way most companies approach buying market research these days. Although this shift has ushered in valid, digitized solutions that cut delivery times dramatically, it has also led to an overabundance of “fast and cheap” survey tools, particularly those fueled by do-it-yourself (DIY) research platforms.
We understand the appeal of these platforms from a convenience and cost standpoint. When executed well, they have potential to be game changers, allowing businesses to be more agile in their decision making.
But, a word of caution: Speed is valuable, but only if the data has integrity. In fact, data is meaningless if you do not have a reliable and valid way to collect and interpret it.
When it comes to predictive analytic surveys, there are many substandard research practices promising fast results. One such practice is collecting data from multiple panels and treating them all the same, or building database norms by simply throwing raw data from multiple sources into a database.
As the R&D outlined in Figure 7 shows, it certainly would not be appropriate to put scores for all of these methods into the same database without accounting for the inherent differences in scores they generate.
Similarly, the data fluctuations displayed in the R&D in Figure 8 underscore the importance of utilizing valid research methodologies and controls. Sadly, many DIY survey platform designers are not experienced in developing questionnaires or sourcing panelists, nor do they have the expertise to understand and manage the variables that can affect overstatement levels. Alternatively, they may choose not to focus on these things at all and put the burden of data management, data quality and sample design practices onto their customers. When this is the case, important factors that affect data validity can be missed.
Finally, to feel confident using survey results for decision making, you need R&D to understand what the data means and to empirically prove that it predicts in-market outcomes. Doing this effectively requires effort, but it also benefits from having a lot of experience and data at your disposal. Getting valid insights is not as easy as simply fielding a survey and collecting responses. Making decisions faster can be a competitive advantage—but only if the decisions being made are rooted in good research and data interpretation.
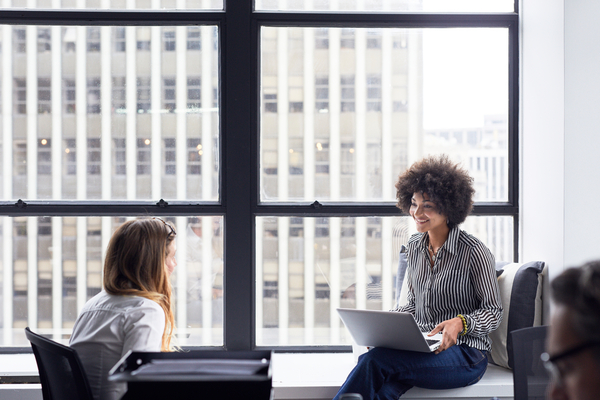
Bringing research in-house?
Those who choose to bring research in-house in order to save time and/or money should determine whether the sample is constructed in a way to provide a reliable answer to the core strategic question. If the core objective is predictive analytics or measuring iterative improvements, researchers should consider the following questions:
- Do they really understand how the sample is being sourced?
- Do they know whether the sample is being managed for data consistency?
- Are they developing their benchmarks and norms in a way that accounts for overstatement differences across panels?
Don’t be faked out of making good decisions
Any discussion about the credibility of survey-based research would be incomplete without addressing the issue of fraudulent data.
To be blunt: The number of “fake” respondents in market research surveys is on the rise and, in some situations, comprises a substantial portion of the data being collected. This battle isn’t new or a surprise to the industry—after all, research participants are typically compensated for the time they invest in taking surveys and, wherever there is a chance to earn money, fraudulent activity is a possibility.
We believe there are two main factors driving this rise. The first is evolving technology that allows fraudsters to subvert traditional fraud detection systems and enables survey bots and digital survey farm fraudsters to complete large quantities of surveys and earn rewards very efficiently. The second is the aforementioned pressure for faster and cheaper insights that, in our opinion, encourages data suppliers and research companies to gloss over data quality problems and not work hard enough to keep up with evolving fraud techniques, ultimately prioritizing quantity over quality.
Sensing the increase in fraudulent survey responses, a brand-led coalition called CASE4Quality was formed to investigate and address this industry-wide problem. The CASE4Quality team consists of senior insights professionals at some of the world’s top consumer products manufacturers, as well as unbiased experts on data supply and sampling insights. This team leveraged four of the primary fraud detections services used in the industry, as well as trap questions and manual reviews to conduct an analysis of data quality across eight major survey sample suppliers.
Their analysis concluded that 30-40% of respondents in their study provided low-quality data, with ~10% being caught by fraud detection tools and an additional 20-30% being identified in reviews of open-end verbatims or responses to trap questions. The “bad” data could be a result of fraudulent respondents, real but disengaged respondents, or a combination of both. Regardless, making decisions off this type of data is dangerous—and businesses deserve better.
CASE4QUALITY Key Findings
Although a portion of the respondents providing bad data in the CASE4Quality investigation would typically get blocked by fraud detection tools in real-world projects—and fraud detection tools can also produce false positives—the result of the analysis reveals two key points:
• Attempts at survey fraud are pervasive.
• As the data in Figures 9 and 10 suggests, much of the bad data is going undetected, poisoning the information that companies are relying on to make million- or even billion-dollar decisions.
At BASES, we have been on top of this challenge, making investments in people, processes and new tools to combat survey fraud attempts. However, fraud detection tools and thresholds are not used in a consistent way across all companies. These findings should be concerning to anyone leveraging survey-based research for their decision making: Subpar data undermines credibility and factors into perceptions that survey responses do not lead to valid, predictive insights.
Retaking control of data quality
Quality survey responses lead to valid insights. The aforementioned quality issues should not be a condemnation of survey research itself: Fraudulent activity can be contained; it just takes the right oversight, focus and diligence.
Three best practices to combat survey fraud
These are the best practices we employ in our own survey data collection, in addition to applying our own tools and quality reviews versus simply relying on the sample provider to do so.
1
Sample providers and market research companies must employ more than one fraud detection tool
2
Additional checks (trap questions) and reviews of the open ends and other data for inconsistencies or illogical responses should be part of any survey response system
3
Ongoing monitoring of data at a system level and constant evolution of techniques is required to stay ahead of the evolving methods of committing fraud
We recommend that anyone purchasing research follows a few best practices to protect the integrity of their data. This is particularly true for those leveraging DIY platforms, as there is no agency side analyst to review data quality and, in some cases, multiple protection tools could be absent. DIY users should consider investing in their own fraud detection resources to layer on top of the tools their research vendor purports to have. They should also develop expertise to catch fraud through manual reviews and trap questions.
Yes, implementing these quality checks may be inconvenient or counter-intuitive to conducting research faster and more cheaply — but if a substantial portion of your survey data is illegitimate, you will simply be paying less money to more quickly make ill-informed decisions. How much will this cost in the long term?
The resiliency of survey research done right
The book Everybody Lies asserts two key points:
- People lie in surveys
- Big data can help uncover the truth
We agree. These principles are, in fact, what our entire new product evaluation system is built on. We have become experts at translating what consumers say into what they do: All of our predictive models are based on linking what people said into a survey and calibrating that information to big data — in our case, purchasing behavior in-market through literally millions of transactional data points. And, as one of the largest survey-based companies, as well as the leader in monitoring sales transactions, we at NielsenIQ BASES are uniquely positioned to do this.
Ultimately, the validity of survey research done right has been very resilient. We contend that effectively managed survey research is just as predictive now as it was 30 years ago. This is evident in the relationship of claims to in-market behavior. Though a lot has changed about consumers and the way they shop and consume media, the relationship between consumers’ survey claims and actual purchase behavior has remained consistent (see Figure 12).
Similarly, the durability of survey research is evident in our sales forecast accuracy. We recently validated BASES sales forecasts for over 200 products launched in the past five years. This analysis showed that our forecast accuracy today (within 9% of actual sales) is that same as it was in the 1980s and 1990s. This consistency can be accomplished only with the fundamental validity of survey research, the proper sample management techniques discussed in this article and ongoing model developments to keep up with the ever-changing and increasingly complex media and retail environments.
While not always perceived this way, survey research also is very flexible in its ability to glean predictive insights in all kinds of situations — from traditional CPG products, to consumer durables, to new-to-world products. Although we often hear that people cannot really tell us how they would react to a truly ground-breaking, innovative product in a survey, our experience disputes that.
We have successfully forecasted sales for everything from one of the first smart phones to category-creating CPG products, all using consumer feedback from surveys as our foundation.
The search for a better way
Although we are firm believers in survey research done right, we acknowledge the ever-changing marketplace and the pressures to move faster. We are also aware of the advancements in technology and the increasing access to data and the opportunities these bring.
We ourselves have responded to these challenges by making dramatic advancements in the speed of our traditional services, employing machine-learning techniques and becoming industry leaders in revolutionary techniques like neuroscience and System 1 research. Survey-based research may be a core building block of BASES’ solutions, but we are always open to and on the lookout for new approaches, provided they can bring something of value for our clients — namely, improved accuracy or faster or cheaper insights — without sacrificing data quality.
In part two of this series, we will discuss some of the new (and old) methods and techniques being leveraged as alternatives or enhancements to survey research, what you can expect from them and how to employ them the right way. Visit and bookmark our BASES thought leadership hub for our forthcoming release, or contact one of our BASES representatives to learn more about our Concept Screening Suite and other solutions.
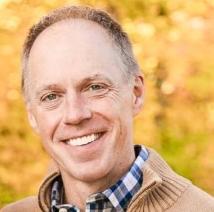
About the author
Mike Asche is a seasoned market research leader with over two decades of experience working on survey-based research. As part of the BASES division of NielsenIQ, Mike has experience in all aspect of market research including data collection, data analysis, client consulting and the development of new market research products and methodologies. Currently, Mike leads the function responsible for data supply and data quality at BASES and is passionate about ensuring that methodologies deliver accurate and predictive results. He is dedicated to championing improvements in data quality across the industry.
The author would like to thank the following contributors to this article: Mason Smith, Sarah Peterson and Eric Merrill; as well as Efrain Ribeiro, Tia Maurer, Carrie Campbell, Mary Beth Weber and the CASE4Quality team.