Generative AI for product innovation: Creative hack or hype?
Suppose you’re a manufacturer seeking a new package design for your eco-friendly dish soap. You task your team with researching current packaging pain points and ideating on creative design elements to solve them, resulting in a few ideas with strong potential.
However, one of your team members decides to uplevel her ideation process with Generative AI (GenAI). Using ChatGPT, she develops a prompt inclusive of those pre-identified packaging pain points along with sustainable packaging trends, asking the large language model (LLM) to draw inspiration from other product categories—like ice cream, breakfast cereal, and condiments. The result? An infinite number of relevant combinations that your team can iterate upon until they strike gold.
As the buzz surrounding GenAI intensifies, many CPG manufacturers are exploring its potential for product innovation. The latest LLMs show great promise, with their ability to understand and interpret natural language, generate huge amounts of content, and even “think” critically. LLMs have applications across the entire spectrum of bringing new products to market; however, given their capacity to scan vast amounts of data to generate concepts using unique category combinations, idea generation might be one of the most exciting.
“Breakthrough innovations often sit at the intersection of great ideas colliding across categories—like the iPhone, a revolutionary combination of computer, phone, camera, and MP3 player that changed how we communicate. It’s these moments of disruption that have the potential to transform entire industries and deliver value to consumers.
When used effectively, generative AI has the potential to accelerate innovation cycles, pulling from a vast repository of cross-category insights that help manufacturers come up with their next breakthrough even faster. This could lead to the rapid development of transformational products that we can’t even fathom today.”— Ramon Melgarejo, Managing Director, NIQ BASES and Advanced Analytics
But don’t expect LLMs to generate groundbreaking ideas on their own. They rely on both the data they are trained on and refined prompts that can generate the desired output. In order to leverage LLMs effectively—and avoid a future dystopia of generic product innovations—innovators must still leverage foundational research to elicit differentiated, consumer-relevant ideas.
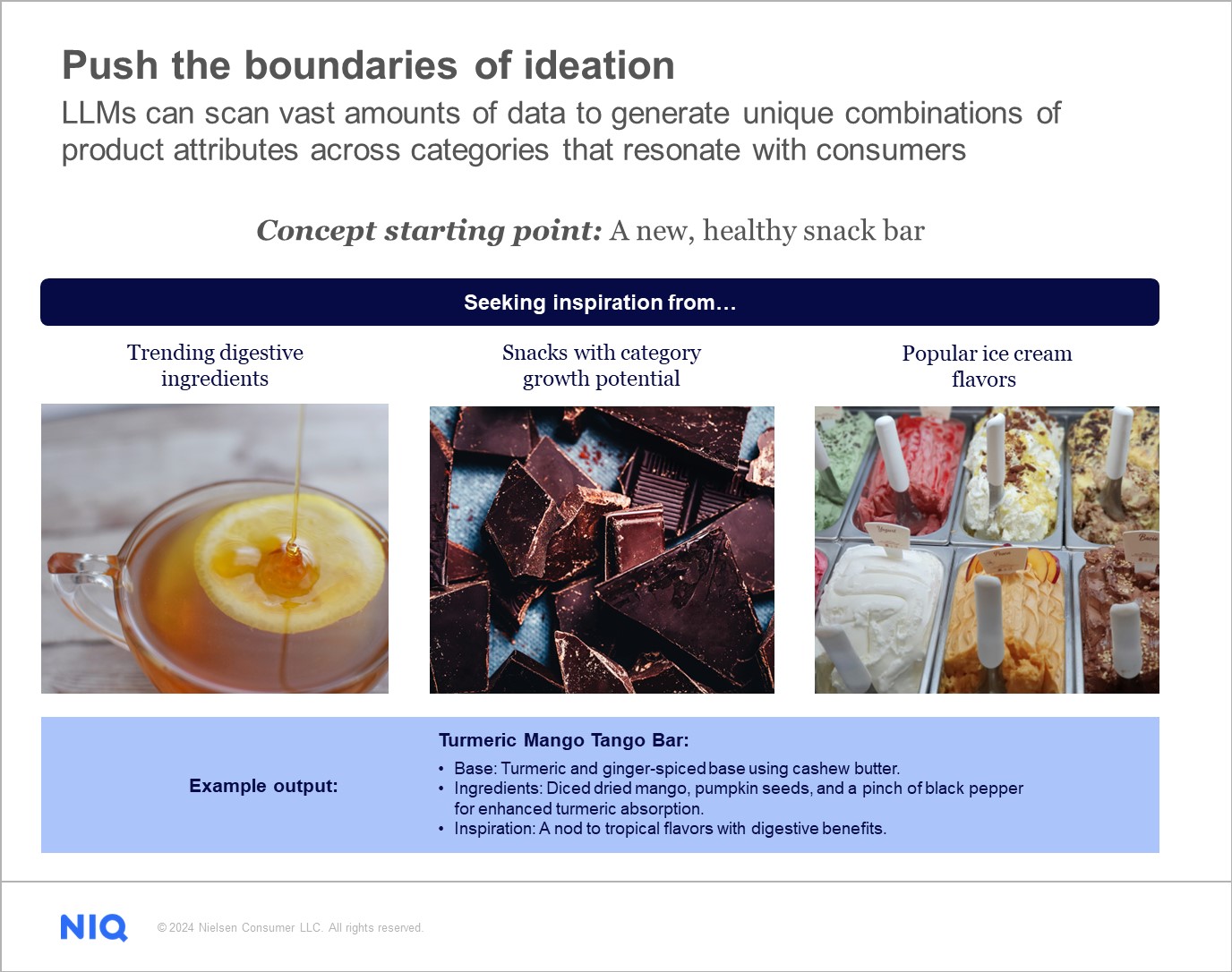
Foundational research: Still the cornerstone of product innovation
An open-source LLM like ChatGPT can easily scour its database to identify consumer trends or popular product attributes. But can you trust the accuracy of that information? Would you bank your business outcomes on it?
Whether your creative ideation relies on man or machine, a solid concept begins with solid research. And when it comes to market research, human expertise still vastly outperforms even the best GenAI models. BASES has shown that cutting research corners comes with a cost, and GenAI brings additional unique risks like bias and hallucinations. Without validation, you cannot be sure whether the trending ingredients identified in your output are backed by current in-market data or are simply part of last year’s viral TikTok challenge.
Furthermore, utilizing foundational research empowers differentiated concepts that are brand and consumer relevant. Inputting details like category knowledge, label insights, or even competitor intelligence can make the difference between generating a generic concept already in play, or a truly breakthrough proposition that requires some iteration.
Spark creative product innovation through rich prompting
Although LLMs can be fine-tuned with any type of data, the rapidly evolving nature of this technology—and, potentially, your own datasets—means it might be more time- and cost-effective to leverage rich prompts inclusive of your research, instead. Rich prompts provide specific details and instruction in the prompt itself, meaning that, as data is updated, the user can simply adjust the prompts instead of retraining the entire model. LLMs are highly capable of managing detailed and iterative prompts, which often produce better and more useful outcomes.
To build effective prompts, keep these guidelines top of mind:
Start with the contextual background
Consider including in your prompt the country, category, and brand knowledge that will improve the relevancy of the output.
Be clear on what you’re trying to achieve
What output do you ultimately want the LLM to provide? Are you looking for new growth levers (e.g. new users, categories, ingredients)? Ideas to renovate a current product? Or something else entirely?
Spark different triggers for creative new ideas
Approach the task from multiple angles to arrive at differentiated, or even unexpected, output (e.g., “Share 2 ideas for each of the personas” or “Borrow ideas from personal care to inspire new innovations for each of these growth goals.”
Regardless of how you utilize GenAI in your product innovation processes, be aware that some of these steps require sharing your own ideas and strategy. Avoid using models that will share this info with the supplier or utilize it for future model training. Also keep in mind that—for now—GenAI is a tool that can augment, not replace, human expertise. Use it for inspiration and ideation as opposed to generating fully baked ideas.
The pace at which companies are adopting GenAI to improve business practices shows no sign of slowing down. The CPG industry is no different. Manufacturers that can successfully wield GenAI’s product innovation potential will find themselves at the forefront of the industry—while those who neglect to embrace it as part of their toolkit will find themselves racing to catch up.
Many thanks to our contributors:
Julie Dellert, Lauren Hirs, Aritra Kanjilal, Martin Levanti, Kamal Malek, Noelle Salerno, Courtenay Verret